Shopping for clothes online has become increasingly popular, offering convenience, variety, and often better pricing than traditional brick-and-mortar stores. However, purchasing clothing online can come with challenges, such as incorrect sizing, quality that doesn’t match expectations, or the hassle of returns. Knowing how to navigate these potential issues can make online shopping more enjoyable and successful. Here are some expert tips to help you buy clothes online with confidence.
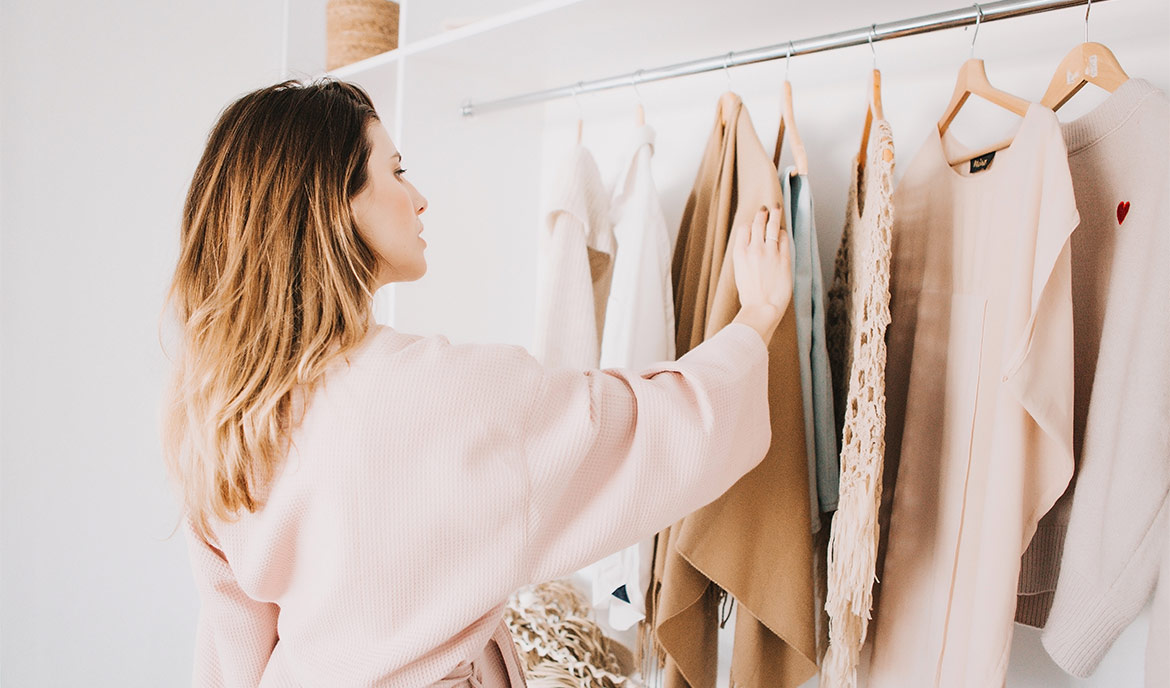
Understanding Size Charts and Fit
One of the biggest challenges of online shopping is getting the right fit. Unlike shopping in a physical store, you can’t try items on before buying, so understanding the size and fit of a garment is crucial.
Measure Yourself Properly
Take accurate measurements of your body to compare with size charts on the website. Common measurements you should know include chest, waist, hips, and inseam. Use a flexible tape measure to get precise measurements, and note … Read more